Current research portfolio
Find below, a summary of some of the research projects on which we are currently working...
Core research projects
This project will build fundamental tools to aid in the deployment and qualification of inspections involving ML in the processing chain through three complimentary but independent work packages. The first work package addresses the use of ML for the suppression of benign responses from component geometry in NDE measurements. The second work package concerns uncertainty in ML algorithms for NDE data. Given any input, an ML algorithm will produce an output, but the confidence and accuracy of that output are currently not well understood or quantified. We will develop techniques that can quantify uncertainty in NDE applications by giving an estimate of the error of output and flag when an algorithm is presented with data that is outside its defined domain of operation. The final work package will explore how ML algorithms for NDE can be made interpretable for end-users by producing a human-understandable description of how a result was reached. This is a key step in the path to qualification of ML-based approaches. The outcomes of this project will be fundamental developments in ML for NDE that will be generalisable to many techniques and will build a solid foundation on which future real world inspections may be developed.
Prof. Paul Wilcox, Ultrasonics and Non-Destructive Testing Group, University of Bristol; Prof. Anthony Croxford, Ultrasonics and Non-Destructive Testing Group, University of Bristol.
We will develop automated UT data analysis tools to improve the reliability of detection, sizing, and characterisation of defects which occur in high safety significance industrial plant. The project will develop capability for defects of critical industrial importance, targeting two species: Thermal Fatigue (a service-induced species) and Hydrogen cracking (a manufacturing/welding defect). Both species are known to occur and often materially impact plant availability/longevity. This project will also enable capability for other challenging defect species going forward, such as stress corrosion cracks.
Dr Stewart Haslinger, Department of Mathematical Sciences, University of Liverpool; Dr Daniel Colquitt, Applied Mathematics, University of Liverpool; Dr William Christian, Department of Mechanical, Materials and Aerospace Engineering, University of Liverpool; Professor Jason Ralph, Department of Electrical Engineering and Electronics, University of Liverpool; Mr. Michael Wright, Signal processing, University of Liverpool; Prof. Michael Lowe, Department of Mechanical Engineering, Imperial College London; Dr Peter Huthwaite, NDE Group, Imperial College London.
In this proposed research work the benefit of the inspection/monitoring mix for different scenarios of degradation and measurement capability will be explored. The amount by which the intervals can be stretched relies on the measurement errors in both the inspection and monitoring method and the spatial and temporal characteristics of the degradation. Therefore, the key challenge to address is to quantify the effect of measurement errors in the process so that probability of detecting and tracking damage can be quantified and spatial coverage and repeat scan intervals can be optimised. In order to do this we will first build a statistical model that simulates the spatio-temporal degradation, based on Kuniewski’s work [3]. We will use this model as ground truth and perturb it to simulate measurements containing errors that are expected in real measurements, e.g. spatial offsets and biases and random noise. Similarly, we will use estimates from experiments and previous work to impose trending errors on the data. Finally, we will use the simulated measurement results to analyse the performance of a pure inspection strategy, or a pure monitoring strategy and mixed strategies and inspection intervals that should be used to get the best results.
[3] S. Kuniewski, “Sampling inspection for the evaluation of time-dependent reliability of deteriorating structures,” Proceedings of the European Safety and Reliability Conference 2007, ESREL 2007 – Risk, Reliability and Societal Safety, vol. 1, Jan. 2007.Dr. Frederic Cegla, NDE group, Mechanical Engineering Department, Imperial College London
Most engineering metals are microscopically polycrystalline, and their manufacturing processes result in the ever-present grain microstructures in the finished products. These include preferred crystallographic orientations (texture), and the so-called macrozones in titanium alloys. These microstructures have been found to be the culprit of many catastrophic failures (e.g. airplane motor explosions), but they are very difficult to detect during the manufacturing process or on the finished components. This project aims to develop an ultrasonic method for the characterisation of the microstructures. It is based on a recent advancement of an ultrasonic measurement of volumetric texture, and will focus on the following areas of work: 1) Automation of the immersion data acquisition equipment. This will expand the existing IP and significantly reduce the data acquisition time in the lab. 2) Physical understanding of shear wave attenuation, which can potentially enable the estimation of statistical geometries in 3D using the same immersion setup for texture measurement. 3) Interpretation of bulk attenuation and backscattering data to obtain microstructure information from normal-incident scans. 4) Exploration of the diffuse wave field methods to apply the characterisation capabilities on complex geometries.
Dr Bo Lan, Non-Destructive Evaluation Group, Department of Mechanical Engineering, Imperial College London
Producing realistic ultrasonic data is vital for a variety of NDE applications in industry: validating methods, testing trainee inspectors, evaluating new methods being developed and generating machine learning data. Numerical methods, such as Pogo (www.pogo.software), have shown strong promise for generating physically accurate data, but suffer from this data missing physical imperfections and noise, as well as often being computationally demanding to produce in the quantities needed, despite the ability to use high-speed hardware such as GPUs. This is a notable challenge for applications such as inspection qualification where many realisations are required across the parametric space of different defects, geometrical considerations etc. This project aims to develop techniques to enable ultrasonic NDE methods to produce necessary data quickly and reliably. The project has three objectives: 1) Develop methods to combine datasets together to generate accurate data at high speed, such as inserting a defect response into another dataset. 2) Develop interpolation techniques to enable finer sampling to be produced from coarse simulations. 3) Develop suitable sampling strategies for high-dimensional parametric space, by eliminating dependent parameters and enabling global performance to be assessed with few simulations.
Dr Peter Huthwaite, Department of Mechanical Engineering, Imperial College London
Impact enhancement projects
The current industrial needs for 3D, volumetric ultrasonic imaging are mainly covered through the use of transducers and transducer arrays. However, transducers have certain drawbacks and limitations: a) it is a contact technique, b) they require couplant, c) they have a considerable size/weight/footprint which may be prohibitive in places with restricted access and e) their delicate electrical connections and packaging may not withstand extreme environments. In short, they are not suitable for remote imaging. The existing project (Remote, 3D ultrasonic imaging in extreme environments using 2D laser induced phased arrays) combines the remote, non-contact nature of laser ultrasonics with the modern paradigms of FMC and TFM in ultrasonics in order to synthesise remote, couplant-free 2D Laser Induced Phased Arrays (2D LIPA) for volumetric, ultrasonic imaging. The aim is to apply then in extreme environments, for in-line process monitoring or in-service volumetric inspection. The aim of the impact enhancement activities is to demonstrate the industrial relevance of 2D LIPAs. We will collaborate with RCNDE industrial members to identify, design and build samples with surface finish, material and geometry that are challenging to existing NDE methods and interesting to industrial applications. At the end of the impact enhancement (IE) grant we want our system to be able to perform remote volumetric imaging of complex components of industry relevant finish and material. Our aim from all these activities is to boost our chances towards technology transfer of 2D LIPAs and the IE funding will provide the means to creating new industrial networking opportunities. Finally, one of the key outputs will be to identify strengths and limitations of the technique, paving the way for attaining higher TRL levels.
Dr Theodosia Stratoudaki, Department of Electronic & Electrical Engineering, University of Strathclyde; Dr Geo Davis, Centre for Ultrasonic Engineering, University of Strathclyde
Recently completed projects
Current NDE techniques for composites are mostly based on techniques originally used on metallic structures. In-order to increase the usefulness of NDE data, new techniques are required to move beyond simple location and extent estimation towards structural prognosis. Strain-based defect assessments have the potential to be a valuable tool for assessing structural components and obtaining predictions of future performance. The aim of the project is to demonstrate the capability of strain-based defect assessments for predicting the degradation of defective composite components.
Dr William Christian, Structural Materials and Mechanics research group, University of Liverpool
Radiographic tomography data does not work well on many realistic AM components, while traditional image processing tools often require significant operator intervention. Advanced deep learning tools are now becoming a promising alternative that can address both issues, but they rely on the availability of large sets of training data, which is not generally available in AM component inspection. To develop deep learning based automated procedure that can work in a wide range of realistic settings, the efficient simulation of domain specific training data is thus crucial to generate the required quantity of training data. This one year project will investigate efficient simulation approaches to generate realistic tomographic data, looking at how this can be used to train and verify defect detection and classification models.
Dr Thomas Blumensath, µ-VIS X-ray Imaging Centre, University of Southampton
This four year project from the University of Bristol aims to develop a general and scalable methodology for automated NDE data analysis based on data science. With a need to analyse ever-greater volumes of complex data from multiple sensing modalities, it uses the availability of computational power to synthesise large (order 10,000-100,000) sets of realistic training data to make a supervised learning approach to NDE data analysis tractable for the first time.
Prof. Paul Wilcox, Ultrasonics and Non-Destructive Testing Group, University of Bristol
The overall aim of this project is to research approaches for simultaneous thermal and microstructure compensation strategies for in-process additive and fusion welding imaging and inspection. It addresses the challenge posed by in-process ultrasonic volumetric imaging of the upper layers of an additive or welded build; namely the effect on wave velocity from thermal gradients and varying microstructures across the component due to build geometry, density, features, arc power and post-arc time. Ultimately, if not accounted for, these variations can give rise to significant incorrect defect size and position, in the order of millimetres in common metallic materials, and hinder the accuracy and ultimate potential of in-process inspection for real-time defect-free process control.
Dr C.N. MacLeod, Dr K. Tant, Centre for Ultrasonic Engineering, University of Strathclyde
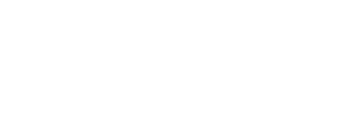
RCNDE – an internationally renowned membership-based industrial-academic collaboration that coordinates research into NDE technologies, ensuring research topics are relevant to the medium to longer-term needs of industry.